topic
jurisdiction
downloadable assets
article
sample
What are the main benefits for lawyers of using Retrieval-Augmented Generation (RAGs)1 and reasoning models2, respectively?
Our research suggests that both RAG and reasoning AI models can enhance the efficiency, clarity, professionalism, and organization of legal work.
Additionally, our findings indicate that RAG models significantly reduce hallucinations in AI-supported outputs, whereas reasoning-based models can enhance the analytical depth of human legal analysis.

Should lawyers prioritise one technology over the other?
This question presents a false choice. Legal technology tools employing RAG are increasingly being built upon reasoning models.
We tested these tools separately both to isolate their individual impacts and because integrated RAG and reasoning-based tools were not available at the time of our experiment.
What are the benefits of combining RAG and reasoning foundation models?
Our findings suggest that RAG models built upon reasoning foundation models may be more effective at identifying the legal source materials most relevant to users' queries.
They may also more clearly articulate the relevance of those materials.
Lastly, these models are likely to be increasingly capable of helping attorneys efficiently enhance their work products across multiple dimensions, including clarity, professionalism, organization, accuracy, and analytical depth.
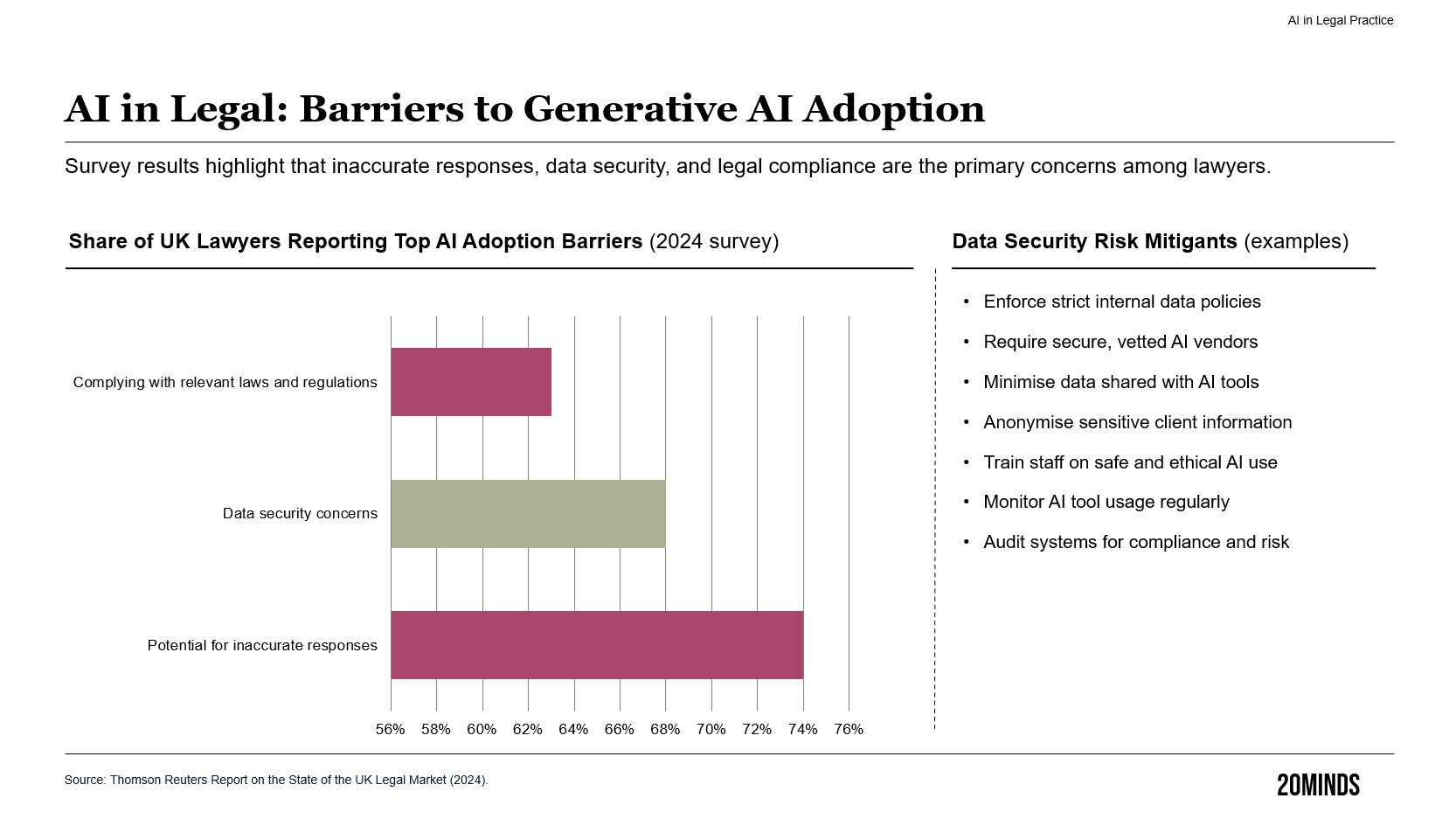
How might malpractice liability standards evolve when errors stem from AI hallucinations rather than failures in human oversight?
I am not convinced that malpractice standards need to adapt at all.
The growing capability of RAG models to reduce hallucinations—both by directly drawing from authentic legal source materials and by enabling users to easily verify these sources—is likely to reinforce awareness that providing fabricated citations constitutes malpractice.
How can buyers of legal services benefit from the advancement of AI?
To fully benefit from AI, legal service professionals must establish systems that enable continuous experimentation with emerging AI tools, alongside careful evaluation of empirical data. Such evaluation should examine both how AI enhances legal work and how it may introduce risks—such as undermining the long-term development of human expertise or encouraging professionals to neglect quality control and analytical rigor. Too many responses by lawyers to AI so far have been driven by empirically unsupported hype or excessive scepticism.
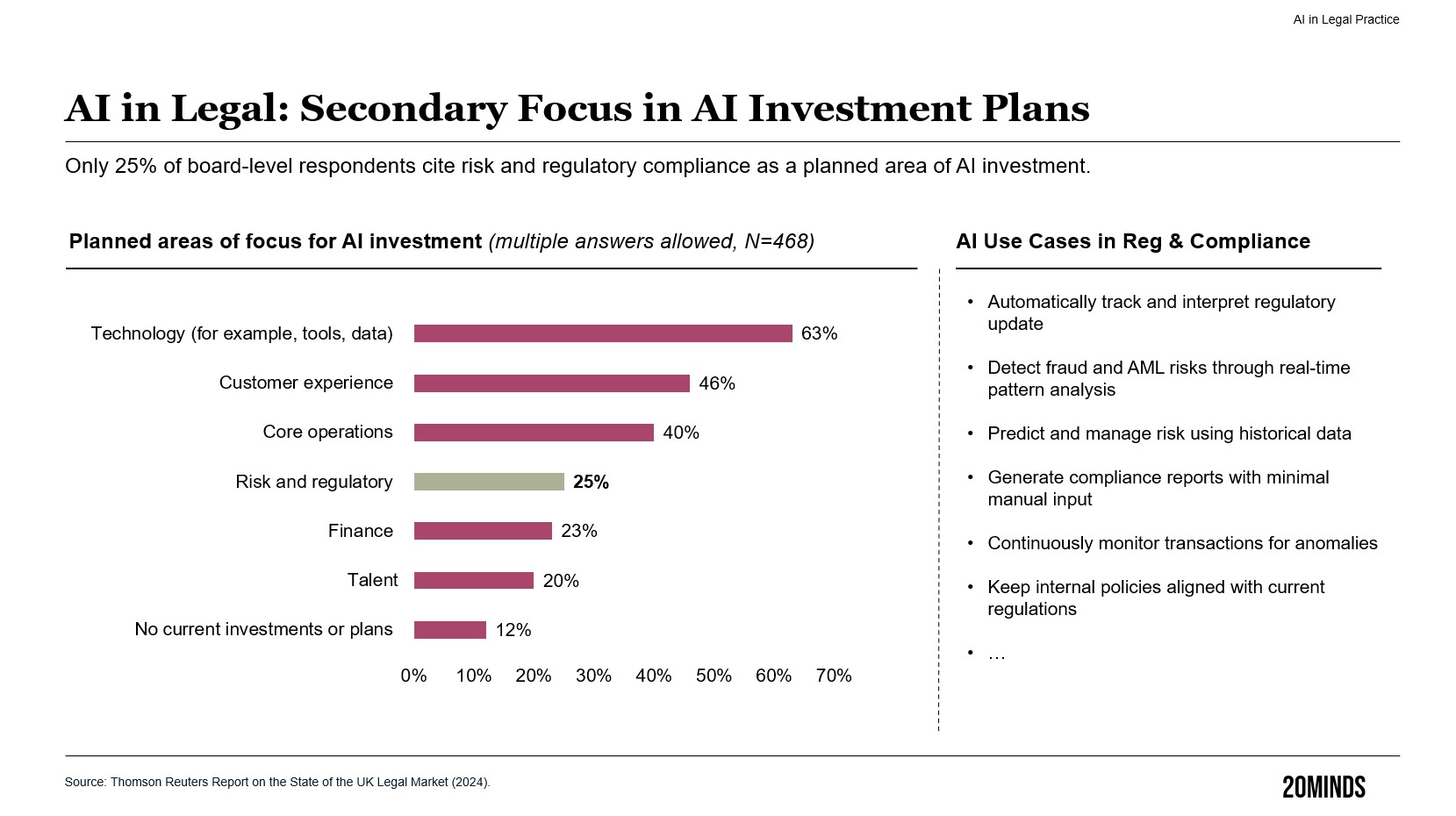
***
Executive Summary: AI-Powered Lawyering: AI Reasoning Models, Retrieval Augmented Generation, and the Future of Legal Practice
By Daniel Schwarcz* (University of Minnesota Law School), Sam Manning* (Centre for the Governance of AI), Patrick Barry (University of Michigan Law School), David R. Cleveland (University of Minnesota Law School), J.J. Prescott (University of Michigan Law School), Beverly Rich (Ogletree Deakins) (*co-first authors)
AI-Powered Lawyering: AI Reasoning Models, Retrieval Augmented Generation, and the Future of Legal Practice (March 02, 2025). Minnesota Legal Studies Research Paper No. 25-16, Available at SSRN: https://ssrn.com/abstract=5162111.
Purpose of Study: First randomized controlled trial evaluating two advanced generative AI technologies—Retrieval Augmented Generation (RAG) and AI reasoning models—in legal tasks.
Tools Tested:
- Vincent AI: RAG-powered legal AI tool grounded in legal sources.
- OpenAI’s o1-preview: AI reasoning model structuring complex legal reasoning before generating output.
- Participants: Upper-level law students assigned six legal tasks using either Vincent, o1-preview, or no AI assistance.
Key Findings:
- Both AI tools significantly improved quality of legal work compared to control group (no AI).
- Productivity gains observed in five of six legal tasks.
- Vincent: 38%–115% improvement.
- o1-preview: 34%–140% improvement.
- Gains particularly strong in complex tasks (e.g. drafting persuasive letters, analyzing complaints).
Accuracy and Hallucinations:
- Vincent: Comparable hallucination rate to control group.
- o1-preview: Produced some hallucinations but delivered higher analytical depth.
Implication: Combining domain-specific RAG capabilities with structured reasoning models may reduce hallucinations and improve legal analysis quality, representing a promising direction for next-generation legal AI tools.
Related publications
Sources
- Retrieval-Augmented Generation (RAG) is an AI framework that improves the accuracy and relevance of responses (“grounding”) by combining a language model with real-time retrieval of information from trusted sources.
- Reasoning models are AI systems designed to simulate structured legal thinking—such as applying rules to facts or weighing competing arguments—making them valuable for tasks like legal analysis, case comparison, or automated decision support.