topic
jurisdiction
downloadable assets
article
Sample
Standards for AI Development and Use
What are the most important standards for AI use and development?
.png)
Kevin: The key standard aspiring to be the global benchmark for AI is ISO/IEC 42001, the AI Management Systems standard. Think of it as the AI equivalent of the Information Security Management standard (ISO 27001), which virtually all larger software providers must be certified with to successfully operate in the market. I strongly believe that within a year or two, most companies using AI are expected to adopt it.
How does ISO/IEC 42001 compare to other standards for AI use and development?
Kevin: ISO/IEC 42001 sets the foundation, but it is part of a broader ecosystem. For instance, it references other ISO standards, like ISO 23894 for AI risk management, which provides more specific guidance on managing risk across the entire AI lifecycle—from data collection and model training to deployment and ongoing monitoring.
Outside ISO (International Organization for Standardization), other significant frameworks exist.
The IEEE provides technical guidance on AI. Its 7000 series covers key issues like algorithmic bias (IEEE 7003), data privacy (IEEE 7002), and ethically-aligned design (IEEE 7000), helping organizations implement responsible AI practices.
Some standards can be audited and certified, while others cannot. Some, like ISO/IEC 42001, are already available, while others, such as ISO/IEC AWI 24028 (focused on AI trust), are still in development.
The NIST AI Risk Management Framework in the U.S. differs from ISO/IEC 42001. It is not certifiable but remains influential due to the dominance of U.S. AI companies. A 2.0 version is already in progress.
.png)
Choosing the Right Standards
These standards seem to overlap. How should an organization pick which ones to follow?
Kevin: Start with the business requirements. Ask yourself:
- How are you involved with AI? If your organization uses or develops AI, a management system like ISO/IEC 42001 is a logical starting point.
- What kind of company are you? Consider your industry, what you are building, and your customers’ expectations. For instance, if you are a healthcare provider dealing with patient diagnostics, you might emphasize data privacy regulations and patient safety standards. If you are a financial services firm developing automated credit-scoring solutions, you may prioritize fairness and explainability requirements for compliance and customer trust.
Then conduct a structured analysis to align standards with your goals:
- Identify Specific Needs: If your products involve ethical or bias challenges—like AI-driven recruiting platforms or facial recognition systems—your management system might highlight the need for additional standards. For example, you may choose an IEEE standard that focuses on reducing algorithmic bias or a sector-specific guideline addressing fairness in automated decision-making.
- Refine with Focused Standards: Use modular standards for more detailed processes. ISO 23894 covers AI risk management by detailing best practices for identifying and mitigating risks throughout the AI lifecycle. IEEE frameworks, on the other hand, offer in-depth technical guidance on topics like transparency, data governance, and system reliability.
- Evaluate Competing Standards: Before committing, compare your options. Standards from ISO, IEEE, or national bodies like NIST can overlap, and implementing them requires resources, time, and training. Conduct a gap analysis to see which standard meets the bulk of your requirements and decide whether you need complementary frameworks to address any remaining areas.
A simple matrix helps map out overlaps between competing standards: For instance, both ISO/IEC 42001 and the NIST AI Risk Management Framework address governance and risk assessment. Comparing these side by side in a matrix highlights similar requirements—like documentation, monitoring, and stakeholder engagement—so you can see where they potentially duplicate efforts.
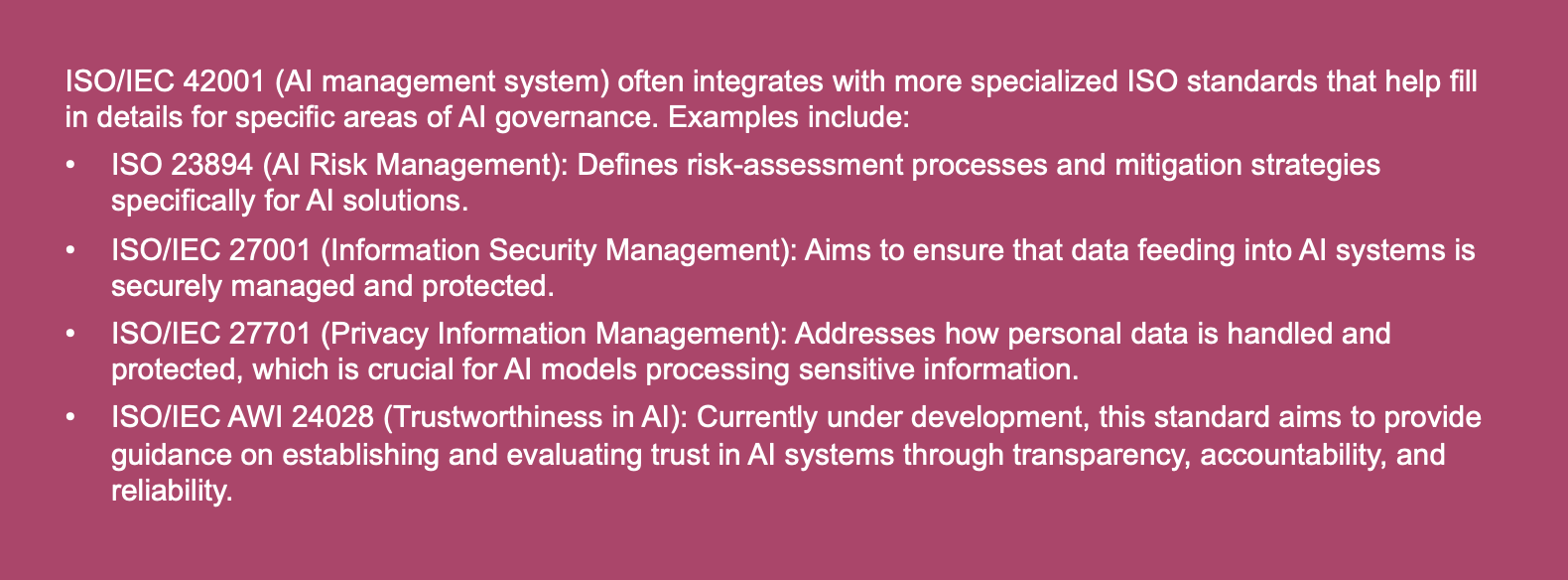
When placed in a matrix, these standards can be mapped according to their focus (e.g., security, privacy, risk, trust) and level of detail (broad management system vs. specialized technical guidance). This makes it easier to see how they can work together—or where they might overlap—to create a cohesive AI governance framework.
The Usefulness of Standards
Implementing management standards can be costly. Why do companies choose to implement management standards?
Kevin: Generally speaking, companies implement management standards to ensure consistent quality of management across their operations. That helps them manage group-wide risks and enhance trust with their stakeholders. Management standards also help streamline processes, reduce transaction and coordination costs, and facilitate interoperability between complex systems, such as when a company is seeking to integrate a new logistics systems or after an M&A transaction.
Companies choose to implement AI management standards specifically for two main reasons:
- A Structured Playbook: Standards like ISO/IEC 42001 serve as a foundational guide—a playbook—for organisations to use AI in a structured, efficient way. They will not make your company an AI governance champion overnight, but they provide the framework for setting up clear processes and structured workflows.
- Future Business Expectation: Certification is rapidly becoming a business necessity. For example, today, software vendors need certifications like ISO 27001 or SOC 2 to prove they handle data securely. Similarly, ISO/IEC 42001 will soon become a baseline for AI-based products and services.
A concrete example: Microsoft has already informed its software vendors that any product containing AI must have ISO/IEC 42001 certification to continue doing business with them. So, this trend is moving quickly.
A market leader acting this way is indeed significant. Are there other examples of companies moving towards this requirement?
Kevin: Yes, major players are already adapting. Recently, Google Cloud Platform (GCP) and Amazon Web Services (AWS) announced that their AI services are ISO/IEC 42001 certified. They believe that their cloud AI businesses depend on this certification. For their customers, having this certification is a critical piece of due diligence to ensure trust and compliance. Standards like these are becoming the de facto trust proxies in AI. Without them, businesses risk losing access to key markets.
Benefits of Certification
What are the benefits of securing certification under the standard versus simply following the standard?
Kevin: Certification offers different advantages:
First, certification positions your organisation as a trusted part of the AI ecosystem. A good analogy is data protection. When you handle personal data, you become part of the data protection chain, with obligations to others in that chain. The same applies to AI. A misconception I often hear is, “We’re just using AI from a respected global vendor, so they’ll handle compliance.” That is simply not true. Every company in the AI supply chain eventually has to comply. Without certification, you risk violating regulations like the EU AI Act.
Certification serves as evidence of compliance, whether to:
- Customers: Certification is stronger than merely claiming to follow a standard. It demonstrates that you have met rigorous requirements, making you a trustworthy partner.
- Regulators or Courts: In the event of a regulatory review or dispute, certification can show that you have adhered to best practices and exercised due diligence in developing or operating AI systems.
Certification Process
Who is involved in the certification process?
Kevin: First, consultants often get involved, although this is not compulsory. They help organisations set up systems, perform gap analyses, and provide advice on achieving alignment with standards. They cannot act as auditors for the same system.
Second, auditors. They are compulsory when you are pursuing certification. Accredited bodies conduct formal assessments to issue certifications. It is essential to ensure your auditor is accredited by a national accreditation body. Some prominent auditors currently offer non-accredited certificates. These do not lead to certifications.
Third, accreditation bodies. National entities oversee the auditors, ensuring they meet the required standards to issue certifications.
Balancing Costs and Return
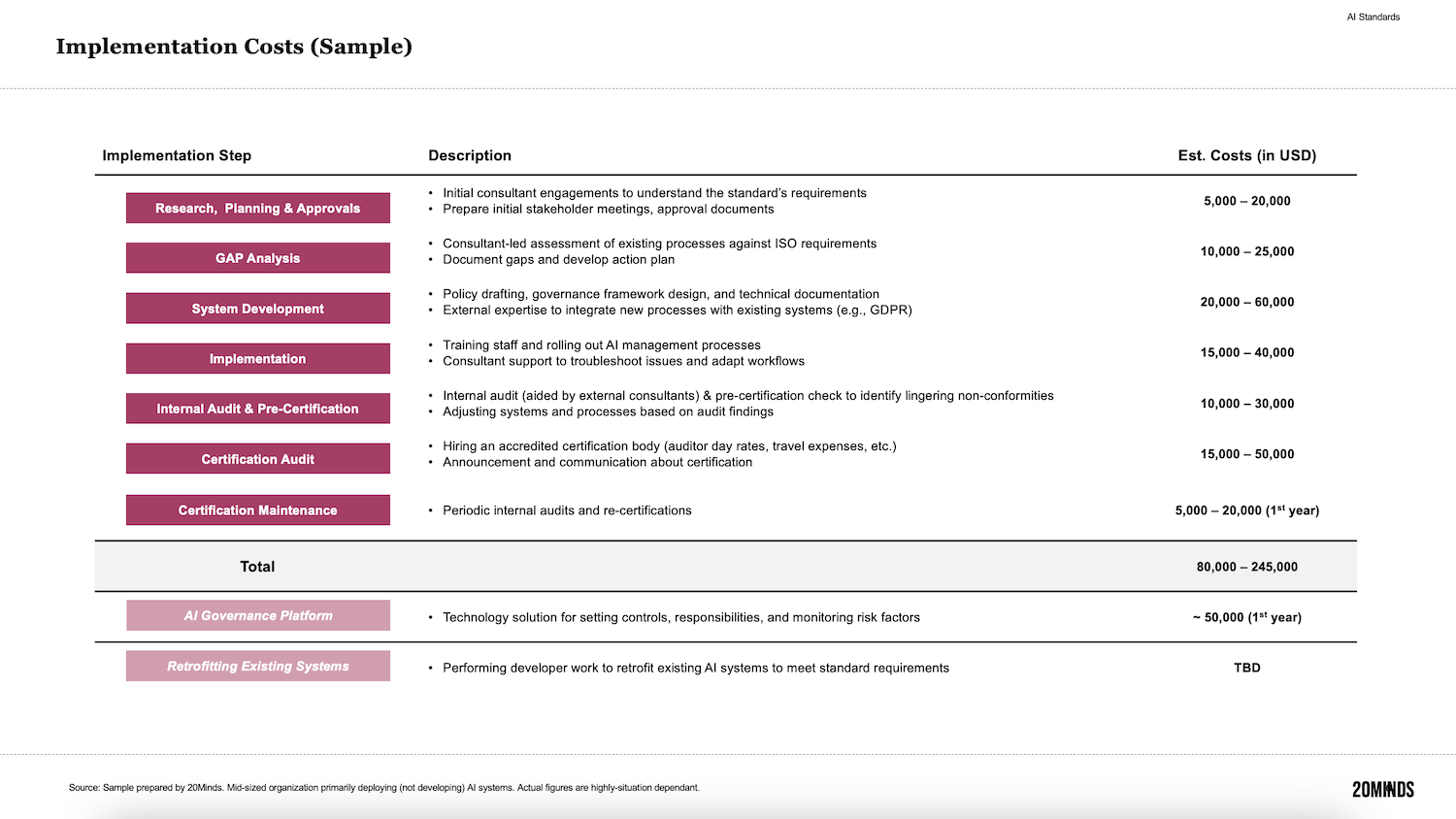
What about costs? Are there synergies with other compliance areas like data protection?
Kevin: Costs involve both setting up and maintaining the system. Maintenance is ongoing and comparable to compliance with data protection regulations like GDPR. While there are synergies between AI management and data protection systems, there are also conflicts.
Example: The EU AI Act may remove some GDPR requirements to reduce bias in AI systems.
Therefore, I recommend separating responsibilities in larger organiations:
- A Data Protection Officer (DPO) should focus on GDPR compliance.
- An AI Officer should oversee AI system operations and compliance with the EU AI Act.
This separation helps manage the inevitable tensions and ensures balanced decision-making within the organisation.
Maturity of AI Standards
Some argue AI standards are immature. With so little real-world experience of companies using AI irresponsibly, how can they be meaningful?
Kevin: This criticism is not unique to standards—it applies to legislation too. The legislative process, like standards development, struggles to keep pace with technological progress. See the EU AI Act. Legislators aim to create future-proof laws, but predicting what tech companies will ship even next year is nearly impossible.
Why not wait until these standards and laws are more established before taking action?
Kevin: That is the worst approach you can take. Legal deadlines, especially for compliance with the EU AI Act, are fast approaching. Larger organisations, particularly those with complex systems, should have started preparations years ago.
For example, some global financial services companies began their EU AI Act compliance journeys two years ago, and many are unsure if they’ll meet the 2026 deadline. Starting now means you’re already behind.
But is not AI Act compliance separate from certification under standards like ISO/IEC 42001?
Kevin: Not really—I see them as interconnected. Many actions required for certification align with EU AI Act compliance. Building a robust AI management system prepares you for both certification and legal conformity. Delaying either process increases the risk of falling short as deadlines approach.
Justifying High Implementation Costs
Implementing standards is not cheap. Fees are spent on consulting and certification—often with intangible returns. How can corporate leadership justify making these investments
Kevin: As said before, companies in the AI value chain ultimately need to comply with a wide set of regulations anyway. By implementing standards, organisations address both customer expectations and regulatory demands, effectively "shooting two birds with one stone."Implementation costs are justified for several reasons:
First, a data privacy breach or an AI-related mishap can lead to regulatory fines and reputational damage far exceeding the cost of implementing standards.
Example: A payment processor spent US$200,000 on ISO risk controls and later saved US$2 million by preventing a major fraud incident.
Second, standards create structured workflows that identify problems early.
Example: A credit-scoring AI adhering to standards could detect bias during testing, avoiding costly rectifications, reputation management and customer care activities down the road.
Third, certification can unlock new markets and opportunities.
Example: A biotech company with AI solutions might secure international clients who require certified vendors.
Metrics and Value Justification
Many standards lack metrics to prove compliance value. How can companies address that gap?
Kevin: This is true; standards often do not provide ready-made metrics or KPIs (Key Performance Indicators) to track compliance value or system maturity.
To bridge this gap, companies can adopt the following strategies:
First, add KPIs to track specific outcomes.
Examples: 'Time to detect an anomaly', 'Mean time to recovery', 'Percentage reduction in false positives.'
Second, compare AI performance metrics before and after implementing a standard.
Example: An AI-driven HR tool applied ISO/IEC 23894 guidelines, with the expectation that this leads to a ~30-40% reduction in hiring bias incidents.
Third, tailor metrics to your industry and use case.
Example: A healthcare AI could track “correct triage rates” or “patient outcome improvements” as a result of risk-based controls.
Fourth, measure maturity. While standards encourage monitoring system maturity, they do not define how to measure it. Organisations can create internal metrics to assess progress over time, such as “percentage of compliance tasks automated” or “frequency of compliance incidents.”
But, there is a common frustration that laws do not define harms well enough to measure them in any meaningful way.
For instance, while laws like the EU AI Act mandate fairness in AI, they do not specify what fairness means (e.g., equality of opportunity vs equality of outcome). Attempts to discuss this with lawmakers often result in blank stares. A solution to this remains to be seen.
Certification as Evidence of Compliance
How strong is certification as evidence in a regulatory or legal context?
Kevin: We are in uncharted territory. To my knowledge, there has not yet been an AI lawsuit involving an ISO/IEC 42001-certified company. However, the EU AI Act includes a provision stating that if an organisation follows state-of-the-art practices, it may not be held liable if its AI causes damage.
Certification provides a clear way to demonstrate compliance with such practices. For example, a well-functioning, certified management system can show that you did everything expected to mitigate risks and follow the latest standards. It is a proactive measure to reduce exposure and build trust.
Does compliance with standards guarantee conformity with the EU AI Act?
Kevin: Not necessarily—this is a political debate right now. The EU AI Office has criticised ISO/IEC 42001, and there is no definitive decision on whether adhering to it will establish full conformity with the EU AI Act. However, harmonised EU standards - such as CEN-CENELEC -, once adopted, will create a presumption of compliance with the EU AI Act’s basic principles. Until then, aligning with recognised standards like ISO/IEC 42001 provides a strong foundation for both compliance and operational consistency across jurisdictions. We may find more national bodies connecting to the EU’s legislation and ISO/IEC connecting to those national bodies. Implementing this global standard is a practical approach to facilitate conformance to legislation across jurisdictions, even if it does not eliminate all liability risks.
Final Thoughts
Final words for time-constrained leadership teams?
The most important advice I can give is: do not sleep on this.
If you need a high-level playbook for how AI regulation might unfold, look at GDPR. Initially, many thought, “It’s just a privacy law—how hard can it be?” They delegated it to their IT teams, assuming minimal effort was needed. Then reality hit. GDPR turned out to be far more complex, requiring cross-functional efforts, and companies found themselves scrambling against hard deadlines. Fines followed soon after.The EU AI Act will likely follow a similar trajectory. Companies that delay preparation will face significant risks, including compliance failures and missed deadlines.
Pro Tip: Book your accredited auditors early. There are very few auditors currently qualified to assess and certify AI systems under standards like ISO/IEC 42001. If you wait until the last minute, you will find yourself at the back of a long queue. Availability could be years out, and by then, it may be too late.
Kevin Schawinski, an astrophysicist and AI governance expert, is the Co-Founder and CEO of Modulos AG, a Swiss company specializing in AI governance solutions. He serves as a member of the European Commission’s General-Purpose AI Code of Practice Working Group and NIST’s Task Force on Generative AI Profile Template Development and Model Safeguards.